Transport and Dispersion (T&D) and Sensor Data Fusion
Intelligently Combining Numerical Models and Sensor Information to Provide Improved Hazard Prediction and Assessment
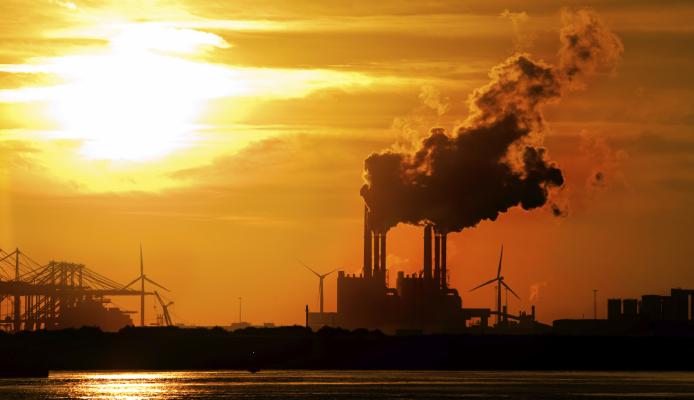
Transport and Dispersion (T&D) and Sensor Data Fusion
Intelligently Combining Numerical Models and Sensor Information to Provide Improved Hazard Prediction and Assessment
Sensor Data Fusion
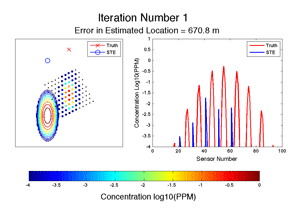
Chemical, Biological (CB), and meteorological sensor systems are being deployed across the globe to protect facilities and personnel against hazardous material released into the atmosphere, both accidentally and intentionally. In the event of a CB agent detection, various questions must be quickly answered, to provide a timely warning to those potentially impacted. These include:
- Where did the material release originate?
- Where is the material heading?
- What concentration/dosage levels can be expected downwind and when?
Unfortunately, information from individual sensors, by themselves, will likely not be sufficient to answer these critical questions. Therefore, the information from all available sensors must be intelligently fused and analyzed to provide a more complete situational picture. Although subjective analysis can be performed by a trained specialist, the time needed to perform the analysis and the potential analysis errors could be unacceptably large. An alternative approach is to develop objective data analysis techniques, which can be performed much more efficiently by a computer system.
This concept of objective analysis is certainly not new to the meteorological community. Objective data analysis and assimilation techniques have been continually developed and improved over the past 50 years, with the primary objective of improving weather–forecast skill. During this time, NCAR has been and continues to be one of the leading contributors to this field of research and development.
NSAP is employing this experience to the CB-hazard prediction problem to:
- provide a more seamless and automatic linkage between real time CB sensor information and operational hazard prediction models
- improve initial conditions for the hazard prediction models, using CB sensor observations
- improve hazard prediction model skill, through the direct assimilation of CB sensor data
An example of this technology, currently being developed for DTRA, is shown in the above figure. This project is utilizing variational data–assimilation techniques and CB–sensor data to provide an estimate of the hazardous–release source characteristics and a refined downwind hazard prediction. The variational technique attempts to minimize the difference between the CB sensor observations and the model predictions, by iterating on the model solution using different initial conditions. It is hoped that this technique will eventually be integrated into operational hazard prediction models such as the Department of Defense's Joint Effects Model (JEM).
Modeling Plumes Of Hazardous Material
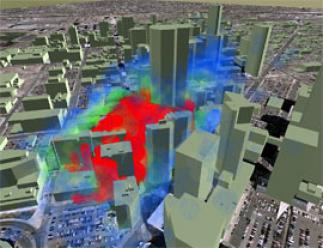
Atmospheric Transport and Dispersion (AT&D) models are utilized for a variety of purposes, including regulatory air quality impact analyses, volcanic–ash–cloud transport prediction, emergency management planning, operational emergency response, and strategic military WMD counter–proliferation efforts. A key ingredient to AT&D modeling is the accurate description and representation of the ambient atmospheric conditions, which drive both the transport speed and the resulting dispersion rates of the airborne material. Although operational atmospheric observation networks can be used, most are unable to resolve the spatial and temporal scales of motion that are essential to AT&D processes. Atmospheric models, combined with these observation networks, can therefore be used to provide a more complete depiction of the driving atmospheric conditions.
In NSAP's efforts to develop, operate, and deploy state of the art numerical weather prediction model–based solutions, it utilizes both operational and research grade Atmospheric Transport and Dispersion (AT&D) models. Operational models, such as Sage Management's Second Order Closure Integrated Puff (SCIPUFF) model and Los Alamos National Laboratory's QUIC model, have been integrated with NSAP's Real Time Four Dimensional Data Assimilation (RTFDDA) model, the Variational Doppler RADAR Assimilation System (VDRAS), and the Variational LIDAR Assimilation System (VLAS) to provide real time analyses and forecasts of hazardous–material plumes. Research grade models, such as NCAR's Lagrangian Particle Dispersion Model (LPDM) and the National Institute for Standards and Technology's CONTAM model have been combined with NCAR's EUlerian LAGrangian (EULAG) Large Eddy Simulator (LES) model to produce realistic synthetic turbulent environments and hazard release scenarios, within which Observing System Simulation Experiments (OSSEs) can be performed. Lastly, NSAP has utilized EPA regulatory models, such as TRC's CALPUFF model, to perform air quality impact studies.
Partners
- U.S. Army Test and Evaluation Command (ATEC)
- Boulder Fire Department
- Defense Threat Reduction Agency (DTRA)
- U.S. Department of Homeland Security (DHS)
- MIT Lincoln Laboratory
- OU, Oklahoma Climatological Survey
Contact
Scott Swerdlin
Director, National Security Applications Program