Artificial Intelligence and Machine Learning
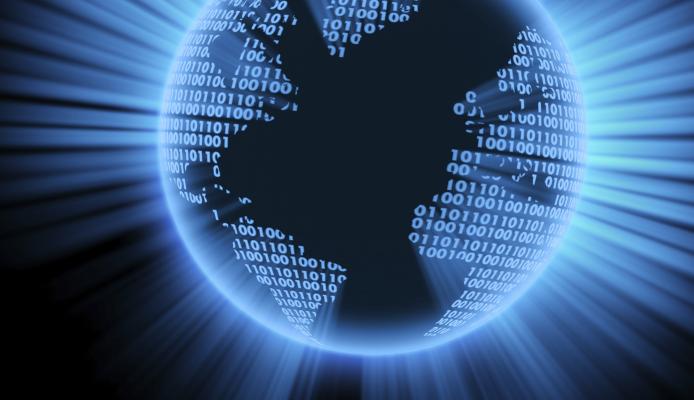
Artificial Intelligence and Machine Learning
Artificial intelligence (AI) and machine learning in earth system science can substantially improve our understanding of the Earth system and climate. As a result, weather prediction models are being improved, with new models combining artificial intelligence and physical modeling. RAL is a leader in the development of intelligent weather prediction systems that blend data from numerical weather prediction models, statistical datasets, real time observations, and human intelligence to optimize forecasts at user– defined locations. The Dynamic Integrated Forecast System (DICast®) and the GRidded Atmospheric Forecast System (GRAFS) are examples of such technology.
DICast® is currently being used by three of the nation's largest commercial weather service companies. Applications of this technology continue to expand as there is a growing desire in industry to have fine–tuned forecasts for specific user–defined locations. This trend is clear in the energy, transportation, agriculture, and location–based service industries. DICast’s impact on renewable energy forecasting has led to its use in other renewable energy projects. In particular, a project with the Kuwait Institute of Scientific Research (KISR) started using DICast® as the core forecast engine for a combined wind and solar forecasting system. This system will combine output from global numerical weather prediction models and a high-resolution version of the Weather Research and Forecasting model (WRF) to produce custom forecasts for an extreme desert climate environment.
In addition, RAL has advanced the application of machine learning to support wildfire prediction. Atmospheric conditions, fuel type, and fuel moisture content (FMC) are critical factors controlling the rate of spread and heat release from wildland fires. Commonly used wildland fire spread models have displaced significant sensitivity to FMC; therefore, having accurate FMC estimates to use as initial conditions is important. RAL is currently building a real-time FMC database to use in WRF-FIRE coupled atmosphere wildland fire prediction model, which is a component of the Colorado Fire Prediction System. The goal is to achieve more accurate accounting for live and dead FMC that will result in more realistic, dynamic representation of fuel heterogeneity and in improved accuracy of wildland fire spread prediction.
These RAL forecast systems also continuing to push the envelope of advanced weather forecasting in the transportation sector. The Maintenance Decision Support System (MDSS) was adapted from its original focus on roadways to be used as a Runway Decision Support System for Denver International Airport (DIA). The system generates tuned weather forecasts and treatment recommendations for the runways at DIA.
Machine learning has proven to be a valuable tool in advanced forecasting technology, and the field is burgeoning with potential. Further advances in road temperature and precipitation forecasts, in predicting gridded fuel moisture content for the U.S. for improved wildland fire prediction, and in improved techniques to wind and solar power predictions will be available to stakeholders in the not-too-distant future.