Uncertainty Quantification and Probabilistic Forecasting
Accurate Point-Based Predictions
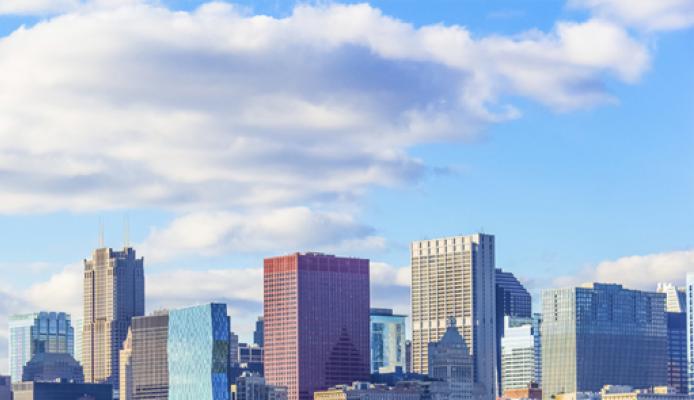
Uncertainty Quantification and Probabilistic Forecasting
Accurate Point-Based Predictions
National security and energy agencies, private businesses such as wind turbine manufacturers and other related organizations need timely, accurate weather and air quality predictions, but also a quantification of their uncertainty. For national security and air quality, reliable uncertainty quantification is central to cost-effective decision-making, for adopting efficient strategies on the ground to protect the public health, and to mitigate the harmful effects of contaminants released either accidentally or deliberately in the atmosphere.
NCAR’s National Security Applications Program (NSAP) has extensive experience with advanced ensemble approaches to generate three-dimensional probabilistic predictions, which include systems based on multi-physics and/or multi-model and/or multi-boundary conditions approaches.
A recent advance in this focus area is the ability to generate accurate point-based predictions and reliable uncertainty quantification at a fraction of the computational cost of traditional ensemble methods, called the Analog Ensemble (AnEn), which has been successfully applied for a range of applications. For example, in a test performed for 0-72 hour predictions of wind power at a wind farm in Italy, the AnEn outperformed a power prediction based on the European Center for Medium range Weather Forecasting (ECMWF) ensemble wind predictions, a worldwide leader in operational forecasting. And, the AnEn computational cost was about one fourth of what was required to generate the ECMWF ensemble.
AnEn has been applied successfully also for the following applications:
- Solar wind power predictions;
- Probabilistic weather predictions over a 2/3D grid;
- Cyclones intensity predictions;
- Air quality predictions of ground-level ozone and surface PM;
- Calibration of ensemble forecasts;
- Wind resource assessment; and
- Downscaling of reanalysis and coarse resolution model estimates.
Partners:
- Army Test and Evaluation Command (ATEC)
- Defense Threat Reduction Agency (DTRA)
- Department of Energy (DOE)
- National Aeronautics and Space Administration (NASA)
-
National Ground Intelligence Center (NGIC)
- National Oceanic and Atmospheric Administration (NOAA)
- National Renewable Energy Laboratory (NREL)
- Vattenfall
- Vestas Wind Systems
- Xcel Energy
Contact
Scott Swerdlin
Director, National Security Applications Program