Noah-Multiparameterization Land Surface Model (Noah-MP® LSM)
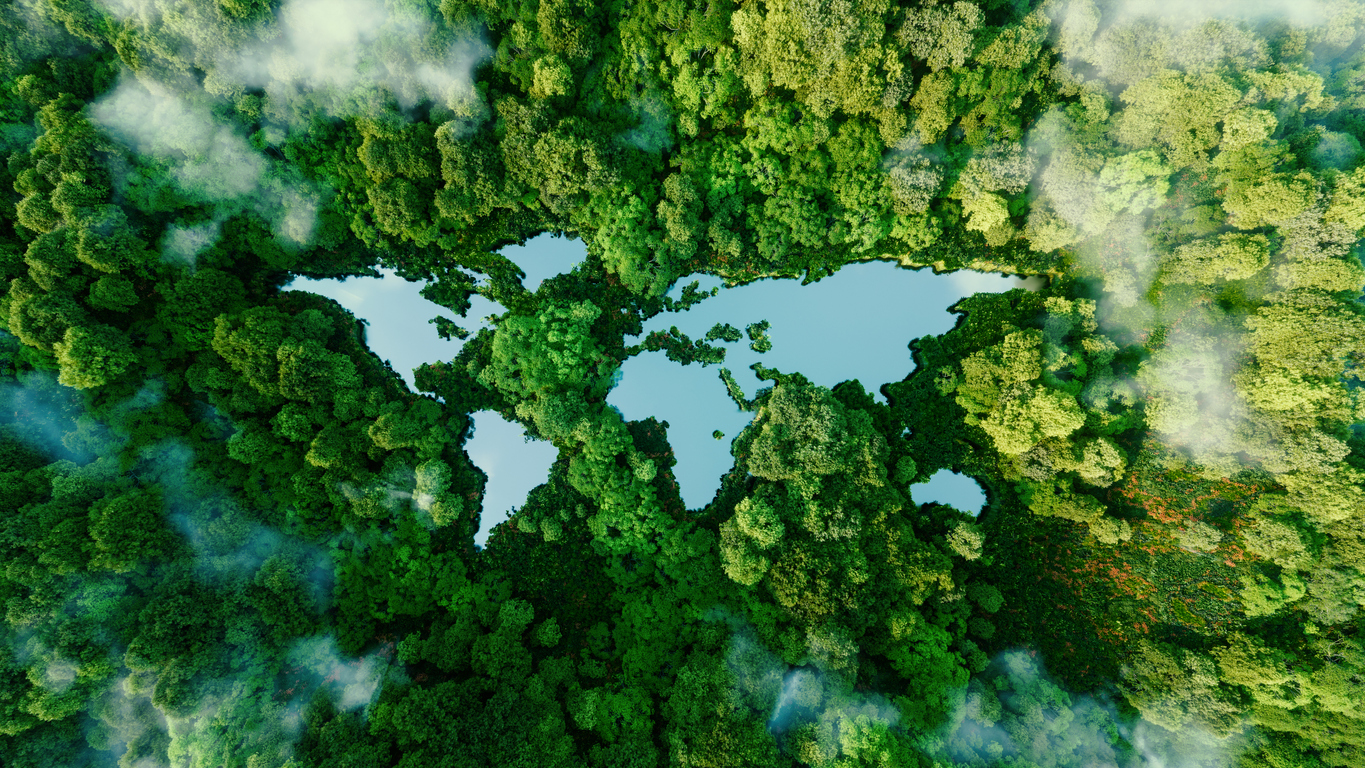
Contents
Overview
Improving the community Noah-MP® LSM to enhance WRF high-resolution climate modeling capabilities
A collaborative effort among NCAR, NCEP, NASA, and university groups has been established to develop and improve the community Noah-MP® LSM, which is a critical component in the operational National Water Model (NWM) and RAL/HAP WRF convection-permitting climate modeling efforts. To enhance its global applicability, the Noah-MP model was evaluated using snow and surface-heat-flux observations obtained from the Colorado Headwaters, Tibetan Plateau in China (Gao et al. 2015), and Boreal Ecosystem Research and Monitoring Sites (BERMS) in Canada (Chen et al. 2015). Based on these evaluations, numerous Noah-MP physics parameterizations have been improved such as canopy snow interception and sublimation for forested regions. New parameterizations of organic soil (Chen et al. 2016), sparse vegetation rhizosphere (Gao et al. 2015), simple nitrogen dynamics (Cai et al. 2016), and a dynamic crop-growth model (Liu et al. 2016) have been introduced to Noah-MP and evaluated against field observations.
Noah-MP® Open-Source Community Land Surface Model. The 4 colors represents: Soil, Water, Vegetation, and Energy. The 4 big circle "C"s represent: Community, Collaborative, Comprehensive, Cutting-edge
Dynamical Downscaling Modeling (DDM) is important to understanding regional climate change and developing local mitigation strategies. The accuracy of DDM depends on the physical processes involved in the regional climate model as well as the forcing datasets derived from global models. We investigated the relative role of LSM and forcing datasets in the DDM over the Tibet Plateau (TP), a region complex in topography and vulnerable to climate change. Three WRF dynamical downscaling simulations configured with two land surface schemes (Noah versus Noah-MP) and two forcing datasets (CCSM vs ERA-Interim) were performed for the 1980 to 2005 period. The downscaled temperature and precipitation were evaluated with observations and inter-compared regarding temporal trends, spatial distributions, and climatology. Results show that the temporal trends of the temperature and precipitation are determined by the forcing datasets, and the forcing dataset with the smallest trend bias performs the best. Relative to the forcing datasets, land surface processes play a more critical role in the DDM over the TP due to the strong heating effects on the atmospheric circulation from a vast area at exceptionally high elevations (Figure). By changing the vertical profiles of temperature in the atmosphere and the horizontal patterns of moisture advection during the monsoon seasons, the land surface schemes significantly regulate the downscaled temperature and precipitation in terms of climatology and spatial patterns (Gao et al. 2016). This study emphasizes that the selection of land surface schemes is of crucial importance in the successful DDM over the TP.
Seasonally (dry and wet) and annualy (ann) averaged observed precipitation (OBS, a0-c0, unit: mm d-1), three simulations (a1-c3), relative biases of simulations (a4-c6, unit: %), and the annual cycle of the monthly precipitation relative bias of the three simulations (d, unit: %) compared to OBS. WRF1: ERA-I with Noah; WRF2: ERA-I with Noah-MP; and WRF3: CCSM with Noah-MP.
Product
Noah-MP is a land surface model (LSM) using multiple options for key land-atmosphere interaction processes (Niu et al., 2011). Noah-MP contains a separate vegetation canopy defined by a canopy top and bottom, crown radius, and leaves with prescribed dimensions, orientation, density, and radiometric properties. The canopy employs a two-stream radiation transfer approach along with shading effects necessary to achieve proper surface energy and water transfer processes including under-canopy snow processes (Dickinson, 1983; Niu and Yang, 2004). Noah-MP contains a multi-layer snow pack with liquid water storage and melt/refreeze capability and a snow-interception model describing loading/unloading, melt/refreeze capability, and sublimation of canopy-intercepted snow (Yang and Niu 2003; Niu and Yang 2004). Multiple options are available for surface water infiltration and runoff and groundwater transfer and storage including water table depth to an unconfined aquifer (Niu et al., 2007).
The Noah-MP model can be executed by prescribing both the horizontal and vertical density of vegetation using either ground- or satellite-based observations. Another available option is for prognostic vegetation growth that combines a Ball-Berry photosynthesis-based stomatal resistance (Ball et al., 1987) with a dynamic vegetation model (Dickinson et al. 1998) that allocates carbon to various parts of vegetation (leaf, stem, wood and root) and soil carbon pools (fast and slow). The model is capable of distinguishing between C3 and C4 photosynthesis pathways and defines vegetation-specific parameters for plant photosynthesis and respiration.
Noah-MP® (and Noah) Run-time Tables for Vegetation and Soil Parameters
-
General parameters
Description
-
Soil Parameters
Description
-
Vegetation Parameters
Description
-
Noah-MP® Parameters
Description
Background Surface Fields
-
USGS 30-second global 24-category vegetation (land-use) data
(26.33 MB)
Description
- readme file
- view graphics
- download data
- program
-
Modified IGBP MODIS 20-category vegetation (land-use) data (ftp)
Description
- The data come from Boston University, with some modifications by NCEP. NCEP has added new categories based on some other data sets, and has remapped certain categories to other indices.
- Information from NCEP (ftp) regarding their recategorization
- Additional information from Boston University
- Download data from NCEP ftp server
- The data come from Boston University, with some modifications by NCEP. NCEP has added new categories based on some other data sets, and has remapped certain categories to other indices.
-
Hybrid STATSGO/FAO (30-second for CONUS /5-minute elsewhere) Soil Texture
(9.2 MB)
Description
- readme file
- Soil categories/textures (zip)
- view graphics [topsoil] [bottom soil]
- download data [topsoil] [bottom soil]
- program
-
1-degree annual mean 2-meter air temperature (used in the model as bottom boundary layer conditions for soil models)
(62.53 KB)
Description
- readme file
- view graphics
- download data
- program
-
NESDIS/NOAA 0.144 degree monthly 5-year climatology green vegetation fraction (ftp)
Description
- Information: See "Surface Fields" on NCEP CPPA/GAPP web site
- Monthly green vegetation fraction data
-
NESDIS/NOAA 0.15 degree monthly 5-year climatology surface albedo (ftp)
Description
- Information: See "Surface Fields" on NCEP CPPA/GAPP web site
- Monthly Albedo data
-
Maximum albedo over snow covered area (ftp)
Description
- Information: See "Surface Fields" on NCEP CPPA/GAPP web site
- More information from NCEP ftp server
- Maximum albedo data
Resources
- AMS 2024 Short Course: Noah-MP Land Surface Model Tutorial
- Noah-MP® Annual Users' Workshops - First annual workshop at NCAR
-
How to Cite: The Community Noah-MP® Land Surface Modeling System Technical Description Version 5.0
Description
He, C., P. Valayamkunnath, M. Barlage, F. Chen, D. Gochis, R. Cabell, T. Schneider, R. Rasmussen, G.-Y. Niu, Z.-L. Yang, D. Niyogi, and M. Ek (2023): The Community Noah-MP Land Surface Modeling System Technical Description Version 5.0, (No. NCAR/TN-575+STR). doi:10.5065/ew8g-yr95
-
Modernizing the open-source community Noah-MP® land surface model (version 5.0) with enhanced modularity, interoperability, and applicability, GMD, to be submitted.
Description
He, C., P. Valayamkunnath, M. Barlage, F. Chen, D. Gochis, R. Cabell, T. Schneider, R. Rasmussen, G.-Y. Niu, Z.-L. Yang, D. Niyogi, and M. Ek (2023): Modernizing the open-source community Noah-MP land surface model (version 5.0) with enhanced modularity, interoperability, and applicability, GMD, to be submitted.
-
Comparisons of Noah-MP® versions: Results from the simple 1D drivers for Bondville
Description
Comparison between Noah-MP 1.1 and 1.0 for Bondville
-
Comparisons of Noah-MP® to Noah: Results from the simple 1D drivers for Bondville
Description
Comparison between Noah-MP 1.1 and Noah 3.4.1 for Bondville
Publications
-
The incorporation of an organic soil layer in the Noah-MP Land Surface Model and its evaluation over a Boreal Aspen Forest
Description
Chen, L., Y. Li, F. Chen, A. Barr, M. Barlage, and B. Wan, 2016: The incorporation of an organic soil layer in the Noah-MP Land Surface Model and its evaluation over a Boreal Aspen Forest. Atmospheric Chemistry and Physics, 16, 8375-8387, https://doi.org/10.5194/gmd-9-1-2016
-
Integration of nitrogen dynamics into the Noah-MP land surface model for climate and environmental predictions
Description
Cai, X., Z.-L. Yang, J. B. Fisher, X. Zhang, M. Barlage, and F. Chen, 2016: Integration of nitrogen dynamics into the Noah-MP land surface model for climate and environmental predictions. Geoscientific Model Development, 9, 1–15, doi:10.5194/gmd-9-1-2016.
-
Quantification of the relative role of land surface processes and large scale forcing in dynamic downscaling over the Tibetan Plateau
Description
Gao, Y., X. Xiao, D. Chen, F. Chen, J. Xu, and Y. Xu, 2016: Quantification of the relative role of land surface processes and large scale forcing in dynamic downscaling over the Tibetan Plateau. Climate Dynamic, doi:10.1007/s00382-016-3168-6.
-
Noah-MP-Crop: Introducing Dynamic Crop Growth in the Noah-MP Land-Surface Model
Description
Liu, X., F. Chen, M. Barlage, G. Zhou, D. Niyogi, 2016: Noah-MP-Crop: Introducing Dynamic Crop Growth in the Noah-MP Land-Surface Model. J. Geophys. Res., DOI: 10.1002/2016JD025597
-
The community Noah land surface model with multiparameterization options (Noah-MP): 1. Model description and evaluation with local-scale measurements
Description
Niu, G.-Y., et al., 2011: The community Noah land surface model with multiparameterization options (Noah-MP): 1. Model description and evaluation with local-scale measurements. J. Geophys. Res., 116, D12109, doi: 10.1029/2010JD015139.
-
The Community Noah Land Surface Model with Multi-Parameterization Options (Noah-MP): 2. Evaluation over Global River Basins
Description
Yang, Z.-L., G.-Y. Niu, K. E. Mitchell, F. Chen, M. B. Ek, M. Barlage, K. Manning, D. Niyogi, M. Tewari, and Y. Xia, 2011: The Community Noah Land Surface Model with Multi-Parameterization Options (Noah-MP): 2. Evaluation over Global River Basins. J. Geophys. Res., doi:10.1029/2010JD015140.
Past Releases
We recommend using the most current version. Support is no longer provided for the following past versions.
Version | Download | Date |
---|---|---|
1.6 | Noah-MP Version 1.6 (as implemented in WRFv3.6) Offline 2D driver code: High-Resolution Land Data Assimilation System (HRLDAS) | Readme | HRLDAS Users' Guide | |
1.1 | Noah-MP Version 1.1 (as implemented in WRFv3.4.1) | Readme | Changes to Noah-MP v1.1 since Noah-MP v1.0 | August 2012 |
1.1 | Offline 1D Noah-MP LSM v1.1 driver program (with sample dataset) | Readme | 02 Oct 2012 |
1.0 | Noah-MP Version 1.0 (as implemented in WRFv3.4) | Readme | April 2012 |
1.0 | Offline 1D Noah-MP LSM v1.0 driver program (with sample dataset) | Readme | April 2012 |