Wildfire Fuel Data Research
Wildfire fuel characterization helps us simulate and predict fire intensity, rate and direction of spread
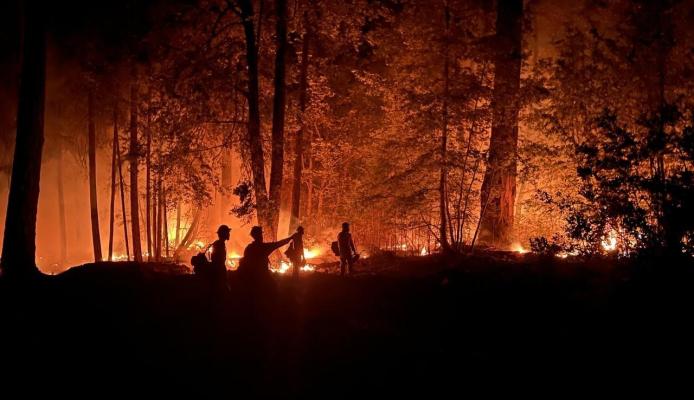
Wildfire Fuel Data Research
Wildfire fuel characterization helps us simulate and predict fire intensity, rate and direction of spread
Wildland Fuel Characterization
We’re developing wildfire behavior models to forecast fire spread (area), direction, and other characteristics helpful for fire suppression and management, fuel treatment (e.g. prescribed burns), and our overall understanding of wildfire behavior. Characteristics that matter include fuel-load depth, percent moisture of extinction, vegetation type, particle size, and heat content. These features are valuable inputs for models to simulate fire behavior. Quantifying fuel characteristics in an accurate and timely way has been one of the main challenges of wildfire mitigation and operational management. These models are only as accurate as the quality of the fuel data we have at the time of need. However, wildfire fuels are dynamic across multiple time scales, from their response to hourly atmospheric conditions, such as morning dew moisture, to their physical changes in response to multi-year disturbance events, such as drought or insect outbreaks. Accurate and timely fuel datasets offer foundational tools that may be mindfully adjusted to provide wildfire behavior models with updated fuel information.
The challenge is to update fuel datasets, in real time, with skill and precision.When used as input for wildland fire behavior models, outdated fuel datasets can cause misleading forecasts, which have negative implications for operational firefighting, mitigation, and wildland fire research. Disturbance events can happen at a temporal scale much faster than wildland fire fuel data are typically updated. The solution may be remote sensing and machine-learning methods to improve on-demand fuel estimation, thus improving fire-prediction skill to protect lives and property.
Advancing Wildland Fire Forecasting - NCAR | RAL
RAL Cindy Halley Gotway
Partners
- NOAA
Representative Projects
- The Dead and live fuel moisture content (FMC) retrievals over the Contiguous U.S. and Alaska Project will use the Weather Research and Forecasting model with fire behavior extensions (WRF-Fire) to quantify the value of the FMC products for predicting fire spread and smoke transport and dispersion.
- LEAP-HI Project aims to develop a unique system for centralizing detailed assessments of wildland fire risk, alerting residents and firefighters days to weeks in advance of the potential for a major fire, RAL's assignment in this effort is combining satellite imagery of land surfaces with highly detailed weather forecasts. These data will be fed into WRF-Fire, which will help identify areas most at risk from blazes.
Publications
-
A Computationally Efficient Method for Updating Fuel Inputs for Wildfire Behavior Models Using Sentinel Imagery and Random Forest Classification
Description
DeCastro, A.L.; Juliano, T.W.; Kosović, B.; Ebrahimian, H.; Balch, J.K. A Computationally Efficient Method for Updating Fuel Inputs for Wildfire Behavior Models Using Sentinel Imagery and Random Forest Classification. Remote Sens. 2022, 14, 1447. https://doi.org/10.3390/rs14061447
Contact
Stefano Alessandrini
Project Scientist III