WRF-Hydro® Community Spotlight | Dr. Jiali Wang
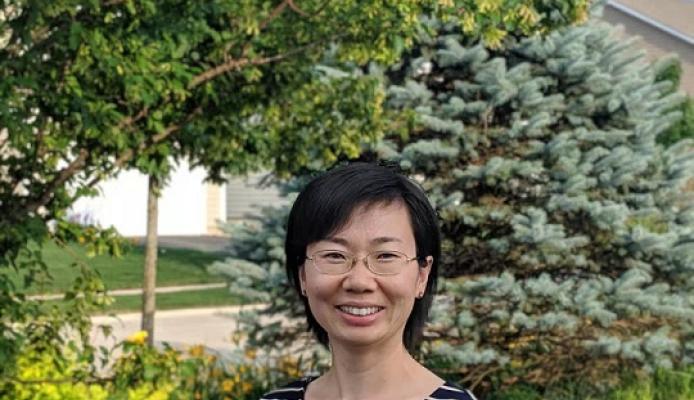
This Community Spotlight focuses on Dr. Jiali Wang and her work on the AT&T climate resiliency study for which she was the primary modeler using WRF-Hydro® and WRF.
>Read the White Paper here<
See our >NEWS page< for links to the NPR Science Friday podcast.
What I have learned from this project is how to effectively transfer the massive dataset to much less amount yet the most important and useful information for the industry partners.
Dr. Jiali Wang is currently an Assistant Atmospheric Scientist in the Environmental Science Division at Argonne National Laboratory. Below is a Q&A with Dr. Wang about her background, experience on the project, and experience with using WRF-Hydro®.
Q: What initially excited you about modeling as your chosen area of study?
A: Numerical modeling is very powerful, although it is not perfect. Using modeling can explore questions that observation data can not do. During my Phd, I investigated urbanization impact on climate (temp; precip) over mega-cities like Beijing. During my postdoc, I investigated global climate warming impact on local weather and climate extremes. For the AT&T project, we look at the global climate change impact on water cycles and wind gust and coastal flooding. Moreover, with the increased size of datasets generated by modeling, there are great opportunities to explore potentials of AI (artificial intelligence). These topics are all exciting to me.
Q: How did you first come to find out about the WRF-Hydro modeling system?
A: I started learning WRF-Hydro in 2016, with a project aiming to understand climate change impacts on the water cycle.
Q: I recall that you attended our WRF-Hydro Hands-on Training in June of 2017. How has that training helped you in working with the modeling system?
A: That was very helpful to learn what physics was still being developed and what setup should be used at the developing stage of WRF-hydro. I also learned that my questions about WRF-Hydro including the GIS tool actually motivated some development of the tool.
Q: How has our community support assisted you in furthering your research with WRF-Hydro?
A: It’s helpful. The website and the documents are also improved a lot and very helpful.
Q: How did you learn about the AT&T project?
A:AT&T wanted to be resilient to climate change, and they want everyone else to be resilient as well. They wanted to know how future water level will change due to heavy precipitation and more frequent flooding/hurricanes; how the wind will change in future, and how all these factors affect their infrastructures. They have done internal and external research and assessments about who to work with to tackle this problem. Argonne was chosen because we had climate model output that are on high resolution and cover the most of North America; we have supercomputing resources which are crucial for numerical simulations we conducted for the project; last but not least, we have a multidisciplinary team with expertise on climate science (including data science and hydrology), statistics, as well as infrastructure modeling and engineering.
Q: Why did you choose to use WRF-HydroⓇ in the AT&T project?
A: WRF-hydro can provide inland flooding water depth (surface head) due to precipitation at a high spatial and temporal resolution. Water depth due to inland flooding is one of the tasks that we conducted for the project. We use 200m resolution for the hydrological component; the input is at 3hour time scale, and our output for streamflow and surface head is at hourly time scale.
Q: What aspects of the WRF-Hydro modeling system made it most suitable for your needs to simulate inland flooding?
A: High spatial resolution down to neighborhood scale.
Q: What were the primary responsibilities and challenges that you had as the primary modeler on the project?
A: In addition to fully cover the WRF-Hydro setup, configuration, simulation; I was responsible for the data management, data check, and data preparation for all the three tasks (inland, coastal, and wind).
For inland flooding, we first interpolate the 12km meteorological variables to 4km, and then run the wrf-hydro at 200m; for the coastal flooding and wind, we work with the original 12km resolution data; since we work with 3 WRF runs driven by 3 different GCMs, there are lots of data to work with. Our timeline was really short, we had kickoff meeting in Mid August, and we had the AT&T team visiting onsite in Mid Oct. We finalized all of our model simulation and analysis by December.Two major challenges were:
1) The output of WRF-Hydro is huge, especially for the RTOUT, which is on a regular grid, while the CHANOUT is point based. One year of data is in the order of TB.
2) How to convert these massive data to numbers that are useful to AT&T?
We conducted generalized extreme value (GEV) analysis for the water depth using all the data we generated for each ensemble members, and estimate the return level of N-year events. We also combine all the members and estimate the uncertainties for future changes of each of these return levels.
For inquiries regarding Jiali's research activities contact jialiwang@anl.gov.
*To nominate a person or a project to be showcased on the WRF-Hydro Community Spotlight please email mollymca@ucar.edu.*