Pioneering better forecasting for UAV operations
RAL partners with NASA's University Leadership Initiative
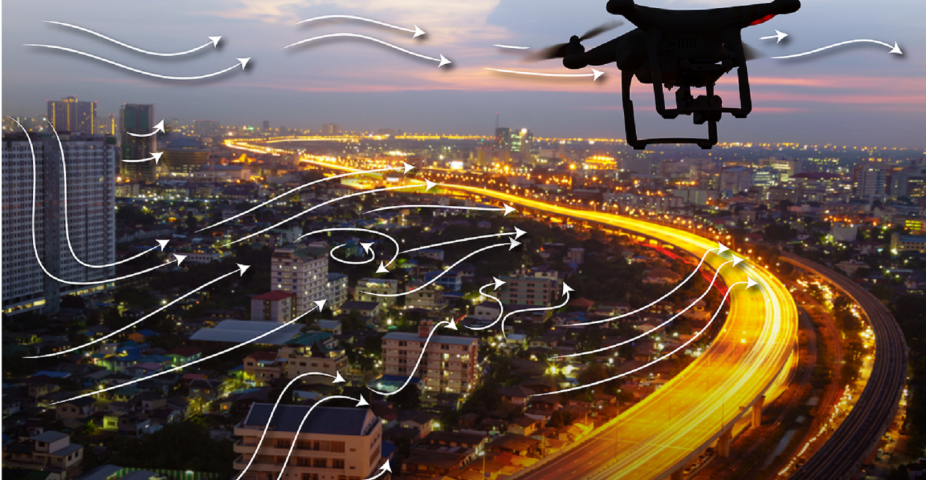
Small Unmanned Aerial Vehicles (UAVs), or drones, are emerging as the next major economic sector of society, as well as a new tool for sensing the lower atmosphere. However, these small autonomous aircraft are much more vulnerable to winds, turbulence and icing than manned aircraft. NCAR studies continue to drive predictive capabilities toward the microscale.
IIn 2018, a team of RAL scientists and engineers developed and demonstrated a system to predict microscale variations in weather to support UAV flight planning during the LAPSE-RATE field experiment. The sub-kilometer predictions of localized terrain-driven flows, wind shear profiles, and turbulent eddies were used to support mission planning and flight operations during the experiment. Participation in this experiment fostered a number of new collaborations with university teams, which ultimately led to a successful NASA (University Leadership Initiative) ULI proposal, kicking off later this year. The overall effort is led by Jamey Jacob at Oklahoma State University and includes researchers and engineers from Universities of Nebraska-Lincoln, Oklahoma and Kentucky as well as Vigilant Aerospace Systems, AirXOS and the Choctaw Nation of Oklahoma.
NCAR’s role in this collaborative initiative is to perform research into coupling UAV-sensed atmospheric data and high-resolution modeling to produce accurate, finescale predictions that are qualified with uncertainty information. There are many challenges to surmount when making sub-kilometer probabilistic predictions of weather in urban areas. This is a budding area of active research NCAR-wide and throughout the world. Plans are to develop testbeds where UAVs will collect observations under a range of weather conditions that will be used to constrain mesoscale flow conditions needed to drive finescale simulations. A newly developed, building-resolving, GPU-accelerated Large Eddy Simulation model called FastEddy® will be used to generate finescale flow fields that represent flows through an urban landscape. Machine learning will be used to relate mesoscale environments to UAV hazards in the flow field produced by FastEddy® and verified by UAV observations. The ultimate goal is to feed this value-added weather information into a UAV Traffic Management System and to demonstrate this new capability in an operational setting by the end of the 4-year project.
NCAR’s effort will be led by James Pinto and Anders Jensen with important contributions expected from Ryan Sobash (MMM), Jeremy Sauer, Domingo Munoz-Esparza, and Sue Dettling. This pioneering convergent research effort will advance aeronautical engineering and atmospheric science research to support an emerging societal need. The safe integration of small UAVs into the National Airspace will require weather guidance at the scales in which small UAV operate. At the same time, UAVs can be part of the solution by collecting observations that feed into operational weather models. Merging cutting-edge atmospheric-sensing technologies developed at our partner universities with mesoscale models and GPU-accelerated FastEddy® LES model, will enable new breakthroughs in quantifying wind flows and turbulence in urban areas under a range of environmental conditions. This is precisely the kind of microscale weather information needed to keep delivery drones flying a safe distance overhead rather than crashing into the side of your home!