Probabilistic Quantitative Precipitation Estimates (QPE)
Timely and accurate Quantitative Precipitation Estimates (QPE) are essential for forecasting stream flow particularly for flash floods and localized urban flooding.
Radar and rain Gauge based QPE is being used in this prediction system for determining:
- How hard it is raining
- How much rain has fallen in the intermediate past
QPE fields are being used in this prediction system:
- As input for quantitative precipitation nowcasting algorithms (Autonowcaster and Trident system)
- As input to the hydrology model (WRF-Hydro)
- For evaluation of radar-based QPE compared to rain gauge measurements of precipitation
- For verification of NWP precipitation forecasts
A variety of QPE techniques are being tested. Details of these techniques can be found at: Radar_QPE_processing.pdf
Model Variability Across U.S. Watersheds
The primary goal is to develop an automated calibration platform for CONUS-wide watershed calibrations. This platform will allow for the quantification of the different sources of uncertainty on streamflow forecast skill.
Skill in model-based hydrologic forecasting depends on the ability to estimate the initial moisture and energy conditions of a watershed, to forecast future weather and climate inputs, and on the quality of the hydrologic model's representation of watershed processes. The impact of these factors on prediction skill varies regionally, seasonally, and by model. We are investigating these influences using a watershed simulation domain that spans the continental US (CONUS), encompassing a broad range of hydroclimatic variation, and that uses the current simulation models of National Weather Service (NWS) streamflow forecasting operations.
Analog Kalman Filter (AnKF)
Two new postprocessing methods are proposed to reduce numerical weather prediction’s systematic and random errors. The first method consists of running a postprocessing algorithm inspired by the Kalman Filter (KF) through an ordered set of analog forecasts rather than a sequence of forecasts in time (ANKF). The analog of a forecast for a given location and time is defined as a past prediction that matches selected features of the current forecast.
The second method is the weighted average of the observations that verified when the 10 best analogs were valid (AN). ANKF and AN are tested for 10-m wind speed predictions from the Weather Research and Forecasting (WRF) model, with observations from 400 surface stations over the western United States for a 6-month period. Both AN and ANKF predict drastic changes in forecast error (e.g., associated with rapid weather regime changes), a feature lacking in KF and a 7-day running-mean correction (7-Day).
The AN almost eliminates the bias of the raw prediction (Raw), while ANKF drastically reduces it with values slightly worse than KF. Both analog-based methods are also able to reduce random errors, therefore improving the predictive skill of Raw. The AN is consistently the best, with average improvements of 10%, 20%, 25%, and 35% with respect to ANKF, KF, 7-Day, and Raw, as measured by centered root-mean-square error, and of 5%, 20%, 25%, and 40%, as measured by rank correlation. Moreover, being a prediction based solely on observations, AN results in an efficient downscaling procedure that eliminates representativeness discrepancies between observations and predictions.
GRAFS (GRidded Atmospheric Forecast System)
Gridded Forecasting
GRAFS (GRidded Atmospheric Forecast System) is a software system that is designed to address the difficult problem of producing high quality weather forecasts at locations where observations are not available. Accurate forecasts at remote locations are used to drive many user–specific applications such as road temperature forecasts along an entire roadway, or soil temperature forecasts for agriculture.
GRAFS combines numerical weather prediction (NWP) model forecasts, statistically refined point forecasts, and climatology to interpolate forecast data to a high resolution grid. Applications using GRAFS typically use 4–km grid cell resolution. Higher resolution grids may be appropriate in regions of rapidly changing topography.
To generate these forecasts, GRAFS starts with model data from a NWP model(s) and then downscales those data onto the target grid using a sophisticated climatological difference interpolation scheme. Finally, this downscaled forecast is corrected to match a statistically optimized forecast generated at a set of observation points.
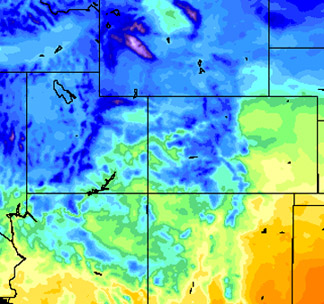
GRAFS technology is ideality suited for downstream systems and users that require precision location–based forecasts. GRAFS is a licensed technology of the UCAR Foundation.
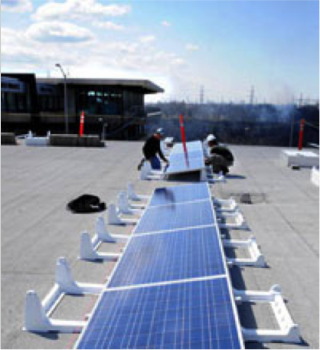
This funding from New York Power Authority is their cost share for the DOE Solar Power Forecasting project. Deliverables are the same as for the DOE project.
We are using these funds to stand up a gridded forecasting system for CONUS. Since it is connected to DOE Solar, the first forecasts will be for solar irradiance.
The Goal is to start a new OpenSource gridded forecasting system that could be used for a multitude of clients in the future
GRAFS - Motivation
- Gridded forecasting system: synthesis of numerical, statistical, and human weather forecasts on a regular grid
- Last mile in information pipeline from forecasters to users
- Problem: current gridded forecasting systems are inadequate, proprietary, or too specialized
- Need: A community gridded forecasting system in which many different interpolation, blending, and correction methods can be tested under a common framework
GRAFS - Vision
- Produce a gridded forecast product that blends information from multiple numerical models with smart artificial intelligence techniques.
- This product will be modular and customizable, allowing a variety of input sources, blending and optimization algorithms, and output formats.
- Make this product widely accessible and applicable for a broad community – work toward joint development and OpenSource software.
- Implement a version that can also contain licensed software and proprietary data.
Dynamic Integrated foreCast (DICast®) System
The Dynamic Integrated foreCast (DICast®) system is tasked with ingesting meteorological data (observations, models, statistical data, climate data, etc.) and producing meteorological forecasts at user defined forecast sites and forecast lead times. In order to achieve this goal, DICast® generates independent forecasts from each of the data sources using a variety of forecasting techniques. A single consensus forecast from the set of individual forecasts is generated at each user-defined forecast site based on a processing method that takes into account the recent skill of each forecast module.
DICast® is a licensed technology of UCAR.
History
The DICast® system was first developed at NCAR in the Fall of 1998 with the goal of generating completely automated, timely, accurate forecasts out to ten days at thousands of international locations. Potential applications of this system include the transportation systems, precision agriculture, and general public-oriented forecasts. See the Operations tab for other future applications of this technology.
The DICast® system ingests data from multiple sources and applies automated forecasting techniques to each data source. Each of these forecast modules produces an "independent" forecast. The forecast skill is then improved using a fuzzy logic scheme to combine the individual forecasts.
Over the years, the numerical weather prediction (NWP) model data used by the system has evolved in response to changes to those models and appearance of new ones. The system is currently capable of using all of the US National Weather Service (NWS) modeling suite (GFS, NAM, RAP, HRRR, ensembles of GFS and CMC) as well as MOS guidance (MEX, MET, MAV, LAMP), European Forecast Center's ECMWF, the UKMET model, Environment Canada's GEM model and the Australian Bureau of Meteorology's ACCESS model. Other models such as high-resolution customized versions of WRF can be added based on the user requirements.
The system is designed to generate forecasts of standard meteorological parameters at a set of user configured locations for each forecast lead time. Forecast extent, forecast interval and update frequency are configurable but generally match the input NWP model temporal parameters. Individual module forecasts are combined using a weighted sum. The weights used in the combination are adjusted daily to reflect the recent performance of the forecast modules.
Future Applications And Development
Future Applications for DICast® Technology
The DICast® system was developed for specific applications in the area of public forecasting; however, the basic technology has many potential applications in the future.
- Spot forecasts for wildland fire support – The DICast® system could be used as a forecast engine for providing temperature, relative humidity, precipitation and wind forecasts at fire weather observation sites that are used as input to the National Fire Danger Rating System.
- Track buckling and separation – The DICast® system could be used to provide air and track temperature forecasts for the railroad industry to be ingested into decision support systems that flag sections of track that are susceptible to buckling and separation due to extreme cold or heat, or rapid changes in temperature.
- Military test range support – The DICast® system could be used to provide surface and upper air forecasts of wind, temperature, precipitation, cloud cover and relative humidity for specified points within military test ranges.
- Hurricane landfall conditions – The DICast® system could be used to provide forecasts for wind, precipitation and storm surge at specified locations along coastlines as a hurricane approaches the coast and makes landfall.
- Marine operations – The DICast® system could be used to provide forecasts for marine buoy and oil rig locations for supporting fishing, recreational boating and commercial marine operations.
- Road Weather – The DICast® technology has been reapplied and configured to generate weather forecasts along specific highway routes as part of the Federal Highway Administrations' winter road Maintenance Decision Support System (MDSS) Project.
- Energy – Because of its ability to tune itself based on local observations, DICast® has proven that it is capable of producing a more accurate forecast than any individual input. This skill can be converted to a significant financial benefit by those responsible for predicting energy demands. The energy industry has expressed a lot of interest in DICast®, particularly for electrical power load forecasting.
Future Development for DICast® Technology
The DICast® system is in a continuous state of development so that it can adapt to new and changing data sources as well as utilize new algorithms. Some of the currently planned major additions include:
- Use the European models (ECMWF, UK Met Office)
- Use NWS digital forecasts
- Create probabilistic forecasts
- Add near-term forecast/obs re-alignment (forward error correction)
Performance
Performance Stats
T = Temperature
Example of RMSE T-Plot
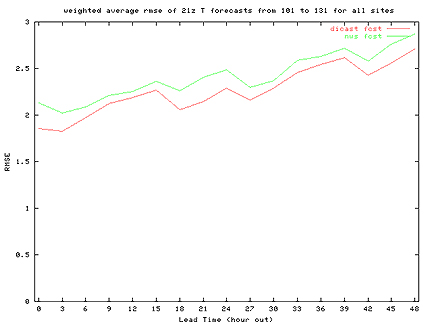
Example of RMSE Max T-Plot
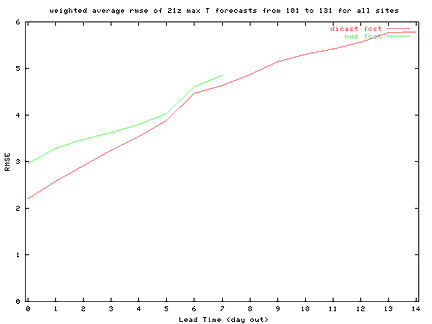
Core Forecasts
The DICast® system generates point forecasts for user–defined locations (e.g., cities, at observation sites, locations along the highway system, agricultural fields, etc.). At observational sites, forecast parameter tuning based on past performance helps improve the forecasts. This class of sites is called core forecast sites. Forecasts at non–core sites are derived from forecasts at core sites.
The numerical weather model data used by DICast® has three–hourly resolution. Since the system is primarily model data driven, forecasts are initially generated at three–hour intervals. These times are called the core forecast lead times.
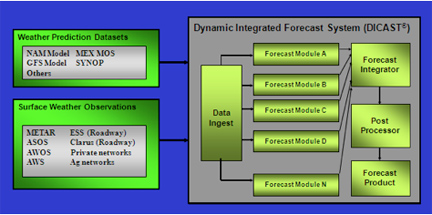
DICast® Forecast Modules
DICast® creates several independent forecast estimates. Each forecast module attempts to create the best forecast it can by applying a specific forecast technique to its input data set. Each DICast® forecast module uses one of three basic techniques to generate forecasts. They are:
- Dynamic Model Output Statistics (DMOS)
- Interpolation of NWS MOS site forecasts
- Semi-static techniques
Each forecast module produces an identically formatted output file. No forecast module is dependent on another forecast module. That is, no forecast module's output is used as input to another forecast module.
Dynamic MOS forecast modules
The Dynamic MOS (DMOS) forecast modules are a dynamic variation of the traditional NWS MOS procedures. DMOS, like traditional MOS, finds relationships between model output data and observations using linear regression methods. However, while MOS equations are calculated using many years of data, DMOS uses only the last 3 months (configurable) of data. New regression equations are re-calculated once per week.
The DMOS technique has several advantages over traditional MOS. The reliance on only a short history allows DMOS equations to be calculated and DMOS forecasts generated for newly ingested models or models that are changing due to enhancements. Traditional MOS equation generation would require the model to be stable (no changes) for several years. Also, the MOS equations are calculated painstakingly with a large human quality control effort. This makes it difficult to add MOS equations for a new set of forecast sites. DMOS forecasts can be made at these sites immediately provided they have an observational history of at least three months (configurable).
A disadvantage of DMOS is that the equations it produces are less stable than MOS equations. For this reason, quality control checks must be put into place to assure that the equations produced will not create nonsensical outlier forecasts.
The DMOS subsystem applied to any model has three components:
- Regressor calculation
- Empirical Relationships Generator
- Forecast Generator
The interaction of these three components is illustrated below:
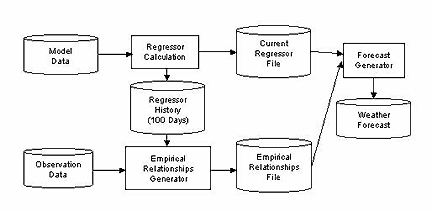
Regressor Calculation
Regressors are variables extracted or derived from model data, which is likely to have a relationship to one of the output forecast variables. These regressors are calculated at each forecast site for each forecast lead-time. About 2/3 of the regressors are variables directly extracted from the model data. Other regressors are derived by combining several variables to estimate meteorological data not explicitly predicted by the models.
Since the forecast sites are rarely at model grid points, interpolation techniques are used to generate forecasts at the forecast sites. This requires an understanding of the projection of the model grid and the terrain assumptions used in each model. As some of the regressors are estimates of meteorological variables at the earth's surface, correcting for the simplified terrain used by the model is important and varies from model to model. The regressors from one model run are all stored in one file. The regressor files are put into a regressor history that the DMOS empirics process uses to calculate regression equations.
DMOS Empirical Relationships Generator
The DMOS Empirical Relationships Generator attempts to find relationships between the regressors and the observations at forecast sites. It does this using a linear regression technique. There are tradeoffs involved in determining the best regression equation. The goodness of fit measure of a regression equation is called its r–squared value. Typically, adding more regressors to an equation increases the r–squared value. However, this also increases the variance of the output forecasts since more regressors are included that do not have a strong relationship to the predictand. Therefore, the desired set of regressors has most of the information leading to a good prediction and does not contain noisy regressors.
Equations that do not have a sufficiently high r–squared value are replaced with a default equation. This default equation is a predefined combination of regressors defined by a meteorologist. A default equation is an attempt to generically replicate a meteorologist's logic in coming up with a forecast. Special, usually derived regressors have been developed for this specific purpose. These default equations generally do not produce the erroneous forecasts that a low r–squared equation might.
This best combination of regressors will vary from site to site, between forecast lead times, and clearly will be different for each forecast variable. The relationships will also vary from season to season and from model to model. The empirics generator is run once per week for each model to find the equations which best fit the most recent data. These equations are stored in a DMOS empirics file and used later by the DMOS forecast generator.
DMOS Forecast Generator
The DMOS Forecast Generator applies the empirical relationships generated by the DMOS Empirical Relationships Generator to the most recent regressors. This generates the DMOS forecast. The relationships between regressors that have done well at predicting the observations recently are used again on today's regressor data to make a DMOS forecast. If any of the regressors that appear in a regression equation are missing, a missing forecast is generated.
NWS MOS Forecast Modules
These forecast modules are based on the MOS products generated by the National Weather Service. The MOS data consist of point forecasts at sites chosen by the NWS. The set of MOS sites is usually a subset of the entire forecast site list. For sites included in any particular NWS MOS forecast, the forecast module tries to reproduce the exact forecast. Where variables are explicitly forecast in the MOS product, they are simply copied. Otherwise, if reasonable, the forecast variable is derived from the MOS data. For some variables, no derivation is reasonable and these variables are left as missing data. If the forecast lead times of the MOS product do not match the system forecast times, the forecast module makes an interpolated forecast where possible.
For many forecast sites no explicit MOS forecasts exist. Forecasts for these sites are generated by interpolation techniques. The interpolated forecasts are generated using the forecasts generated at the MOS sites. No satisfactory interpolation technique has been found that works well for all variables in rough terrain. For example, the interpolation of surface winds in the mountains does not work well using any known technique.
Semi–Static Forecasts
Two forecast modules are called semi–static in that their forecasts depend only on historical data, not on any predictive forecast model. These two are the climatology and persistence forecast modules. These modules look at the past weather over different time ranges and base their forecast on the average weather seen. The climatology forecast module uses data from up to the last 30 years. Monthly averages of the forecast variables have been computed and stored in a climatology file. These monthly climatological values are interpolated to the forecast date. The persistence forecast module averages the observations of the variables seen in recent days to come up with its forecast. The persistence and climatology forecast modules have more effect on the forecasts for longer–term forecasts periods (> 72 hours).
FORECAST INTEGRATION
Integration Overview
The DICast® forecast modules each generate as complete a forecast as possible. This includes a forecast for every forecast variable at every forecast site for every forecast lead time. These independent forecast estimates are combined by the integrator to generate one final consensus forecast. Numerous combination techniques have been developed. Investigation has led to a decision to use an enhanced Widrow–Hoff learning method. This method creates its final forecast using a weighted average of the individual module forecasts. The weights are modified daily by nudging the weights in the gradient direction of the error in weight space. The effect of this is that forecast modules that have been performing well for a particular forecast (variable, site, and lead time) get more weight and the poorly performing modules get less weight. Note that different weight vectors exist for every forecast generation time due to differing latencies in the input data sets. The interaction of components of the integrator is illustrated in the figure below.
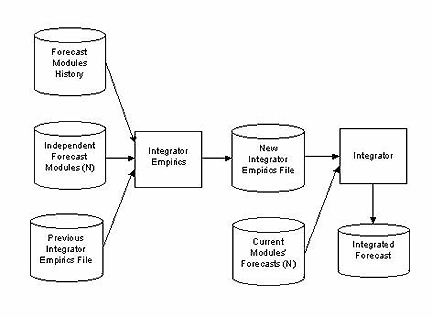
Integrator Empirics
This DICast® process runs once per day and updates all the weights based on the performance of the various forecast modules. It reads the observations from the previous day and compares the forecast modules' output that predicted those observations. For each forecast, the errors are computed and the gradient vector in weight space is computed. A step proportional to the size of the combined error is taken in that gradient direction to compute the new weights.
Integrator
The integrator creates a final forecast by making a bias-corrected confidence–weighted sum of the individual module forecasts. It reads the forecasts from the forecast module output files, the weights from the integrator empirics file, performs its calculations, and stores its results.
Non-verifiable Data Extractor
The DICast® forecasting techniques described above only apply to core forecast variables. These are variables that are regularly measured and reported in meteorological observation data. The DMOS forecast modules and the integrator both require specific observations to tune themselves. The weights used in the combination are pre determined by a meteorologist familiar with the models and stored in a configuration file. The model variables to be combined have been extracted by the DMOS regressor calculation process and stored in a regressor file. The Non–Verifiable Data (NVD) extractor reads in the appropriate models' regressor files along with the weight configuration file before creating its weighted combination output.
Post-processor
The post–processor provides a variety of processing options to merge the integrator's forecasts and the NVD forecasts. It attempts also to remove ridiculous forecasts, derive other forecast variables, and spatially and temporally interpolate the forecasts to non–core forecast sites.
Quality control measures are applied to the integrator's output to ensure that no forecasts are well beyond reasonable ranges. Forecast values near the limits are returned to the bounding values. For example, forecasts of 101% probability of precipitation are turned into forecasts of 100%. Forecasts well beyond the bounds are replaced with a missing data flag.
Forecast variables required by users are derived from the core set of MDSS forecast variables. For example, relative humidity is derived from temperature and dew point temperature. The output of the integrator contains only forecasts for core forecast sites. Forecasts at the non–core sites are generated by spatial interpolation from the core sites' forecasts. Temporal interpolation of the three–hourly forecasts to one hour is used to generate the desired final forecast temporal resolution.
Presentations
A Turbine Hub Height Wind Speed Consensus Forecasting System
Contact
Please direct questions/comments about this page to:
Jim Cowie
Software Engineer/Programmer IV
Soil Condition Analysis & Prediction
A High-Resolution Land-Data Assimilation System (HRLDAS) was developed at NCAR/RAL to provide analysis and prediction of high-resolution soil and vegetation conditions. The advantage of HRLDAS is its use of 1-km-resolution land-cover, soil-texture maps and terrain heights, and 4-km rainfall data. Because of this high-resolution aptitude, it can capture fine-scale heterogeneity at the surface and in the soil.
RAL has been collaborating with industry sectors to develop agricultural decision-support capabilities that optimize the timing of pesticide application and irrigation. These projects typically utilize advanced weather and land-surface models and intelligent data-fusion technologies that continuously optimizes the weather and soil predictions. This research has led to improvements in HRLDAS, the Dynamic, Integrated Forecast System (DICast®), and the Noah Land Surface Model (LSM). This research is instrumental in providing critical input to the weather and land-surface modeling, and satellite communities, and comprises a cross-disciplinary effort. Continued work in this area will lead to more precise prediction of weather and soil condition and more efficient and profitable agricultural operations.
WRF-Crop
RAL has been engaged with the research community to develop an integrated modeling system that captures interactions between weather, climate, crop growth, urbanization, and hydrology. Developing the integrated WRF-Crop modeling system involves two steps: 1) coupling the Noah-MP photosynthesis and soil hydrology components with crop-growth models, and 2) developing crop-specific parameters required by crop-growth models.
The crop-growth models are intended to simulate phenological development, growth and yield formation from emergence until maturity using crop genetic properties, weather, and soil conditions following methods developed and adapted in the agriculture communities. Simulated processes include phenological development rate, gross CO2 assimilation, maintenance respiration, dry matter partitioning for biomass accumulation, growth and senescence of leaves, extension of roots, and crop yields. WRF-Crop incorporates a database on crop characteristics per species, which, for instance, includes the following crop-specific characteristics:
1. Specific leaf area as function of development stage.
2. Emergence: sow date, temperature threshold from sowing to emergence, initial crop dry weight, leaf area index at emergence.
3. CO2 assimilation characteristics of a single leaf: initial light efficiency, rate of net or gross CO2 assimilation at light saturation, respiration in the dark.
4. Conversion efficiency of the sugars produced in the assimilation process into leaves, stems, roots, and grains.
5. Seasonal partitioning of newly formed dry mass into leaves, stems, roots, and grains. Note that the fraction of total dry matter to various plant parts depends on plant development stages.
6. Maintenance respiration rate.
7. Initial rooting depth and maximum rooting depth.
8. Death rate of leaves, roots, and stems.
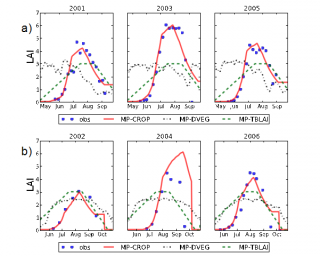
This project aims to improve the representation of cropland-atmosphere interactions in the community Noah-MP LSM with the ultimate goal to integrate it in a coupled model to improve seasonal weather forecasts and regional climate simulations for the NCAR Water System Program. Croplands cover 12.6% of the global land and 19.5% of the continental United States. Through seasonal change in phenology and transpiration, crops can efficiently transfer water vapor from the crop root zones to the atmosphere. Crops have a detectable influence on regional distributions of atmospheric water vapor and temperature, and can affect convective triggering by modifying mesoscale boundaries. Therefore, croplands can significantly influence land-atmosphere coupling, surface exchanges of heat, water vapor, and momentum, which in turn can impact boundary layer growth and mesoscale convergence/convection.
We have introduced dynamic corn (Zea mays) and soybean (Glycine max) growth simulations and field management (e.g., planting date) into Noah-MP and evaluated the enhanced model (Noah-MP-Crop) at field scales using crop biomass datasets, surface heat fluxes, and soil moisture observations. Compared to the generic dynamic vegetation and prescribed-LAI driven methods in Noah-MP, the Noah-MP-Crop showed improved performance in simulating leaf area index (LAI) and crop biomass (Fig. 2). This model is able to capture the seasonal and annual variability of LAI, and to differentiate corn and soybean in peak values of LAI as well as the length of growing seasons. Improved simulations of crop phenology in Noah-MP-Crop led to better surface heat flux simulations, especially in the early period of growing season where current Noah-MP significantly overestimated LAI. The addition of crop yields as model outputs expand the application of Noah-MP-Crop to regional agriculture studies. There are limitations in the use of current GDD criteria to predict growth stages, and it is necessary to develop a new method that combines GDD with other environmental factors, to more accurately define crop growth stages. The capability introduced in Noah-MP allows further crop related studies and development.This new Noah-MP-Crop modeling capability was released in the WRF version 3.7 in 2016. Further test and evaluation are undertaken in the context of coupled WRF regional climate simulations.
Liu, X., F. Chen, M. Barlage, G. Zhou, D. Niyogi, 2016: Noah-MP-Crop: Introducing Dynamic Crop Growth in the Noah-MP Land-Surface Model. J. Geophys. Res., DOI: 10.1002/2016JD025597
Weather Research and Forecasting Model (WRF)
The Weather Research and Forecasting (WRF) Model is a next-generation mesoscale numerical weather prediction system designed to serve both atmospheric research and operational forecasting needs. It features two dynamical cores, a data assimilation system, and a software architecture facilitating parallel computation and system extensibility. The model serves a wide range of meteorological applications across scales from tens of meters to thousands of kilometers. The effort to develop WRF began in the latter part of the 1990's and was a collaborative partnership principally among the National Center for Atmospheric Research (NCAR), the National Oceanic and Atmospheric Administration (represented by the National Centers for Environmental Prediction (NCEP) and the (then) Forecast Systems Laboratory (FSL)), the Air Force Weather Agency (AFWA), the Naval Research Laboratory, the University of Oklahoma, and the Federal Aviation Administration (FAA).
Four-Dimensional Weather (4DWX) System
Objective
To improve meteorological support through the development and application of new technologies.
Description
The Four-Dimensional Weather (4DWX) System is the product of twelve years of research and development sponsored largely by the U.S. Army Test and Evaluation Command (ATEC), and is accredited for operational use at seven test ranges. Through the use of new capabilities in high-resolution mesoscale modeling, short-term thunderstorm prediction, multi-dimensional integrated displays, and fine-scale climatological analysis tools, the ranges now provide test customers with more accurate test go/no-go guidance.
In its original conception, 4DWX was targeted solely for providing advanced capabilities to the ATEC range meteorology branches, but NCAR has since adapted the system and created derivative technologies for use by other organizations:
- Analysis of potential exposure of U.S. troops to nerve agents during the first Gulf War;
- Consequence assessment for counter proliferation mission planning in Afghanistan and Iraq;
- Anti-terrorism support for the 2002 Salt Lake City Olympics, the 2004 Summer Olympics in Athens and the 2006 Winter Olympics in Torino;
- Urban-scale modeling in Washington D.C., and OKC;
- Protection of the Pentagon and surrounding regions; and
- Support for firefighters in CO and AZ during summer wildfires in 2002.
Army Test-Ranges
4DWX was originally developed to provide advanced capabilities solely to the ATEC ranges.
Case Studies
NCAR has adapted 4DWX and created derivative technologies for use by other organizations.
Demos
4DWX products stand alone or can be coupled with other specialized applications.
Sponsor
ATEC | DTC
The U.S. Army Developmental Command (DTC) is the developmental testing arm of the U.S. Army Test and Evaluation Command (ATEC) and the Army's premier organization for conducting developmental testing of weapons and equipment. With the largest, most diverse array of testing capabilities in the Department of Defense , DTC tests military hardware of every description under precise conditions across the full spectrum of arctic, tropical, desert and other natural or controlled environments on highly instrumented ranges and test courses.
Collaborator
DTRA
The Defense Threat Reduction Agency safeguards America and its friends from weapons of mass destruction (chemical, biological, radiological, nuclear and high explosives) by reducing the present threat and preparing for the future threat.
NGIC
The National Ground Intelligence Center provides scientific and technical intelligence (S& TI) and general military intelligence (GMI) on foreign ground forces in support of the warfighting commanders, force and material developers, DA, DOD, and National- level decisionmakers. The NGIC also manages the Army's Foreign Material Exploitation Program and foreign material acquisition requirements and constitutes a single authoritative source for comprehensive ground forces threat to the Army and other services
ATD
The Atmospheric Technology Division develops, provides and operates state-of-the-art, reliable atmospheric observing systems and associated support services to the university-based research community for purposes of climate and weather research worldwide. Facilities include aircraft operation and instrumentation, the deployment of radars, in-situ instruments and arrays, sondes and other electronic sensors in the field, and the development and support of data assimilation and analysis software.
SCD
Scientific Computing Division's goal is to enable the best atmospheric research in the world by providing and advancing high-performance computing technologies. SCD offers computing, research data sets, data storage, networking, and data analysis tools for NCAR users.
MMM
The Mesocsale and Microscale Meteorology Division's primary focus is on understanding atmospheric phenomena on spatial scales ranging from micrometers to megameters and time scales from seconds to a few days.